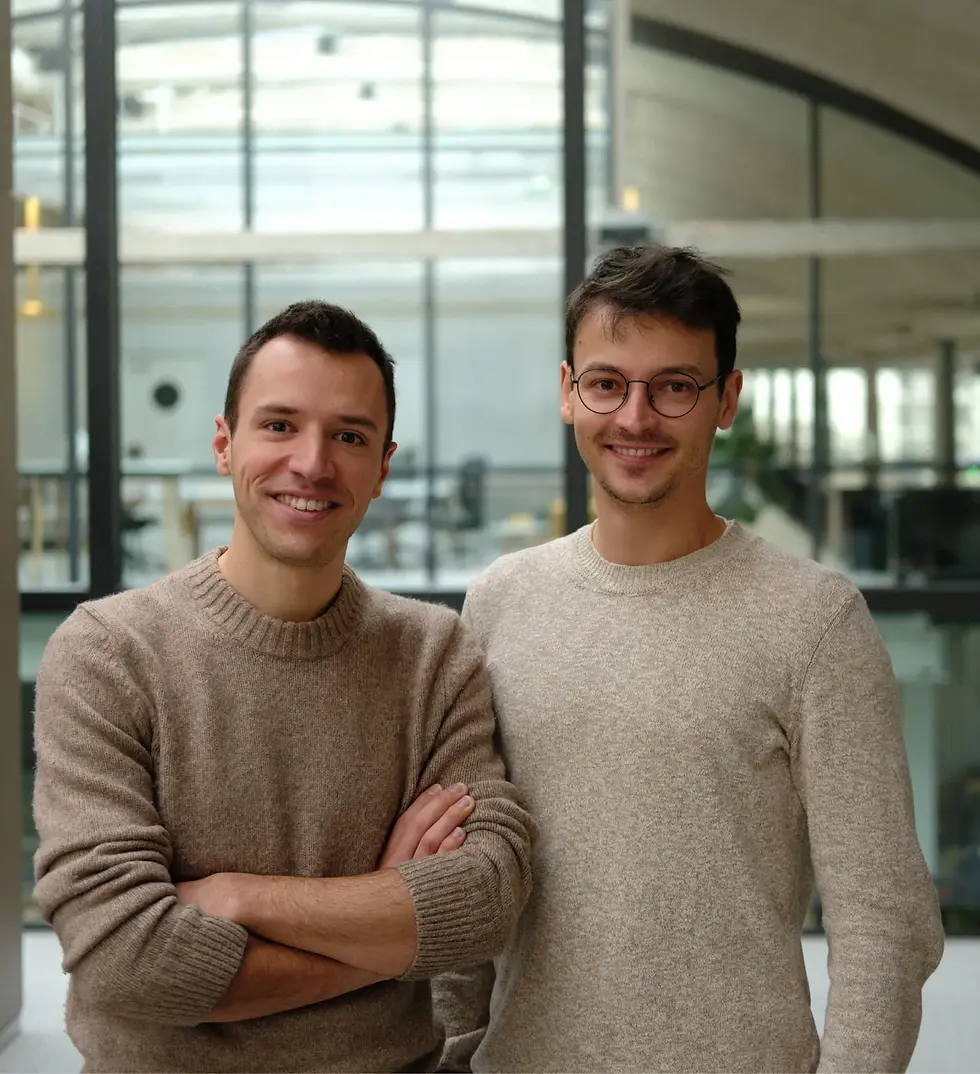
In the realm of artificial intelligence (AI), most innovations have been geared toward unstructured data—text, images, and speech. Large Language Models (LLMs) like GPT-4, Gemini, and Claude have dominated the conversation, excelling in natural language understanding and content generation. However, an often-overlooked form of data—structured tabular data—remains the backbone of industries like finance, retail, logistics, and healthcare.
Neuralk-AI, a French deep-tech startup, is stepping into this gap by developing the first tabular foundation model designed explicitly for structured data. With a $4 million funding round led by Fly Ventures and contributions from investors including Thomas Wolf (Hugging Face), Charles Gorintin (Alan), Philippe Corrot, and Nagi Letaifa (Mirakl), the company aims to transform the way businesses utilize structured datasets.
This article provides an in-depth exploration of the importance of tabular data, the challenges with existing AI approaches, and how Neuralk-AI’s groundbreaking foundation model is set to revolutionize structured data intelligence.
The Power of Tabular Data: The Backbone of Business Intelligence
What is Tabular Data?
Tabular data refers to structured datasets stored in relational databases, spreadsheets, or CSV files. This data is highly organized into rows and columns, making it ideal for analytical processing. Some common examples include:
Customer Databases: Personal information, purchase history, demographics
Product Catalogs: SKU numbers, descriptions, pricing, availability
Financial Records: Transactions, revenue, expenses, profit margins
Logistics & Inventory: Supply chain data, warehousing, stock levels
Healthcare Records: Patient history, medical reports, drug interactions
Unlike unstructured data, which requires semantic interpretation, tabular data enables direct analysis and computation.
Industries That Depend on Tabular Data
Industry | Use Cases of Tabular Data | Challenges with Traditional AI |
Retail & E-commerce | Product recommendations, inventory optimization, demand forecasting | Poor adaptability of LLMs for structured datasets |
Finance & Banking | Risk assessment, fraud detection, credit scoring | Legacy statistical models lack predictive power |
Healthcare | Drug discovery, patient history analysis, medical billing | Privacy concerns and regulatory compliance |
Supply Chain & Logistics | Fleet management, demand-supply analysis, route optimization | Complexity in integrating real-time updates |
Why AI for Structured Data Has Lagged Behind
Despite AI’s rapid advancements, structured data intelligence has not seen the same level of innovation as NLP (Natural Language Processing) or CV (Computer Vision). Current AI models struggle with:
Data heterogeneity: Different structures across businesses create compatibility issues.
Scalability: Existing ML algorithms are limited in handling large, high-velocity datasets.
Computational costs: Deploying AI on structured data can be resource-intensive.
Accuracy & Generalization: Classic ML methods require extensive feature engineering, limiting adaptability.
Alexandre Pasquiou, co-founder and Chief Scientific Officer of Neuralk-AI, emphasizes:
"The data that truly holds value for businesses has been structured for decades. Yet, we still rely on outdated machine learning techniques. Our model modernizes structured data processing, making it as intelligent and adaptable as LLMs for text-based AI."
Neuralk-AI’s Tabular Foundation Model: A New Paradigm
What is a Tabular Foundation Model (TFM)?
Neuralk-AI is pioneering the world’s first tabular foundation model (TFM), designed to learn from structured data at scale. Unlike conventional ML models, which require manual feature engineering, TFMs autonomously extract patterns, relationships, and trends from tabular datasets.
According to Neuralk-AI CEO Antoine Moissenot:
“We’re not adapting LLMs for structured data; we’re building an entirely new architecture that understands tables natively.”
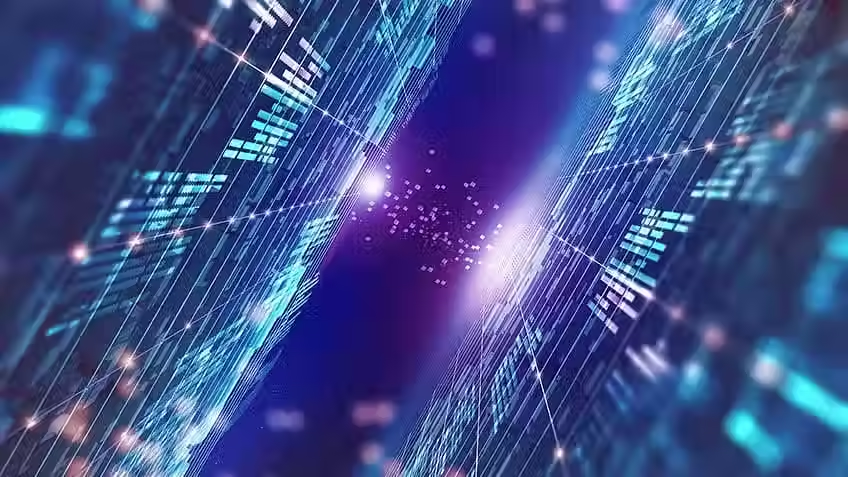
Key Capabilities of Neuralk-AI’s TFM
Feature | Description | Impact on Businesses |
Self-supervised learning | Learns patterns from unlabeled data | Reduces reliance on expensive labeled datasets |
Smart Deduplication & Enrichment | Identifies redundant data and fills missing values | Ensures cleaner, more accurate datasets |
Predictive Analytics | Analyzes trends to optimize decisions | Improves demand forecasting and dynamic pricing |
Fraud Detection | Detects anomalies in financial transactions | Reduces fraud risks in banking & e-commerce |
Enterprise-Grade API | Seamless integration with existing systems | Enables real-time AI-driven decision-making |
Benchmarking Against Existing AI Models
Neuralk-AI plans to release benchmark comparisons in the coming months. Currently, structured data AI is dominated by:
XGBoost & LightGBM: Highly efficient gradient boosting models used in finance and commerce.
DeepTabNet (by Google AI): A deep learning framework optimized for tabular datasets.
TabNet (by Google Brain): A neural network that applies attention mechanisms to tabular data.
Neuralk-AI’s goal is to outperform these models in accuracy, efficiency, and scalability.
The Role of AI in Commerce: How Neuralk-AI is Disrupting Retail
E-commerce and retail industries thrive on data-driven decisions, yet traditional analytics methods lack predictive intelligence. Neuralk-AI’s foundation model enables retailers to:
Optimize Inventory Management – AI-driven demand forecasting prevents overstocking and stockouts.
Enhance Customer Personalization – Hyper-targeted recommendations improve conversion rates.
Detect Fraud in Transactions – Identifies suspicious patterns in customer behavior.
Automate Data Workflows – Reduces manual errors and increases efficiency.
The company has partnered with major French retailers including E.Leclerc, Auchan, Mirakl, and Lucky Cart to deploy its AI model in real-world environments.
The Future of AI in Structured Data: Challenges & Opportunities
Challenges in Scaling AI for Tabular Data
Challenge | Potential Solutions |
Data Privacy & Compliance | Implement GDPR & industry-specific AI ethics |
High Computational Costs | Develop efficient, low-power AI architectures |
Enterprise Adoption Barriers | Educate companies on AI-driven data strategies |
Opportunities for Global Expansion
While Neuralk-AI is starting with commerce, its foundation model has cross-industry potential:
Finance: AI-driven risk modeling for investment firms.
Healthcare: AI-assisted diagnostics from medical records.
Supply Chain: Real-time logistics optimization.
Neuralk-AI plans to expand its offerings by September 2025, establishing itself as the leading AI company for tabular data representation learning.
The Dawn of AI-Driven Structured Data Intelligence
Neuralk-AI is not just another AI startup—it is reshaping the field of structured data intelligence. While LLMs have revolutionized unstructured data processing, the next AI frontier lies in unlocking the full potential of tabular datasets.
As AI continues to evolve, businesses must embrace domain-specific foundation models like Neuralk-AI’s TFM, which are purpose-built for structured data processing. This innovation will lead to smarter, more accurate decision-making across industries.
Stay Ahead with AI Insights
For expert insights on Predictive AI, Big Data, Quantum Computing, and Emerging Technologies, follow Dr. Shahid Masood and the team at 1950.ai. Stay updated on the latest breakthroughs in AI-driven structured data intelligence.
Comments