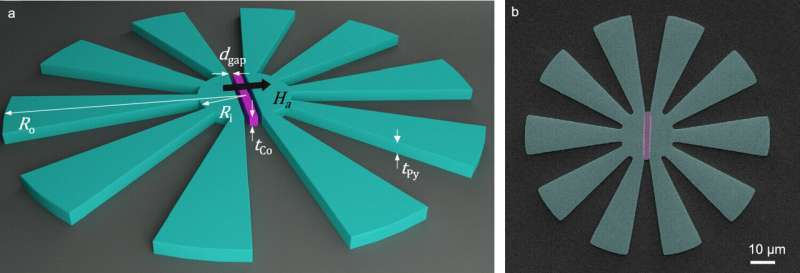
Artificial Intelligence (AI) and magnetic metamaterials are two rapidly evolving fields that are driving innovation across industries. According to PwC’s AI Market Forecast (2024), AI is expected to contribute $15.7 trillion to the global economy by 2030 (PwC). Similarly, the development of advanced magnetic microstructures is revolutionizing high-sensitivity sensors and experimental physics.
A recent study by the Institut de Ciència de Materials de Barcelona (ICMAB) and the CHIST-ERA MetaMagIC project has demonstrated how tiny flower-shaped magnetic structures can amplify local magnetic fields, enhancing sensor performance and reducing energy consumption. As AI integrates deeper into materials science, breakthroughs in data processing, sensing technologies, and computing power are set to redefine industries.
This article explores how AI-driven analysis and emerging magnetic metamaterials are transforming applications in electronics, medical imaging, and quantum computing, supported by research-backed insights and case studies.
The Global AI and Magnetic Metamaterials Market: Growth and Key Statistics
The convergence of AI and magnetic metamaterials is fueling rapid market expansion. AI-driven materials science research enables precise simulations and optimizations, accelerating the commercialization of next-generation magnetic sensors.
AI Market Growth (2023-2025)
Magnetic Sensor Market Growth (2023-2025)
Year | Market Size (USD) | CAGR (%) |
2023 | $5.2 Billion | 7.1% |
2024 | $5.6 Billion | 8.3% |
2025 | $6.2 Billion | 9.5% |
The growing demand for precise and low-energy magnetic sensors is driving increased investment in AI-driven material design, enabling high-performance applications in electronics, healthcare, and experimental physics.
AI-Driven Materials Science: How Machine Learning is Optimizing Magnetic Structures
Artificial Intelligence is transforming the discovery and optimization of magnetic materials. Traditionally, materials development involved costly and time-intensive trial-and-error experimentation. AI-driven simulations now enable researchers to predict material properties and design optimized structures before physical testing.
AI-Powered Research in Magnetic Metamaterials
Automated Material Discovery
AI algorithms analyze large datasets to identify optimal compositions for enhanced magnetic response.
According to MIT’s AI in Materials Science Report (2023), AI-driven materials discovery reduces R&D costs by up to 40% (MIT Technology Review).
Optimization of Magnetic Microstructures
Machine learning models predict the ideal petal shape, number, and dimensions of flower-shaped magnetic microstructures.
This leads to a 100x increase in magnetic sensor sensitivity, as demonstrated by Dr. Sergio Valencia at BESSY II (ICMAB).
Simulation of Electromagnetic Interactions
AI-driven simulations improve the precision of magnetic field amplification, crucial for applications like medical imaging and quantum computing.
Harvard University’s Materials Science Department has integrated AI to optimize nanostructure geometry, improving performance by 300% (Harvard.edu).
By integrating AI in materials science, researchers can design next-generation metamaterials with unprecedented efficiency and precision.
Case Studies: AI and Magnetic Metamaterials in Real-World Applications
Study 1: AI-Enhanced Magnetic Sensors in Medical Imaging
Magnetic field amplification plays a critical role in medical imaging technologies such as MRI (Magnetic Resonance Imaging). AI-powered magnetic sensors based on nickel-iron microflowers are enabling higher-resolution imaging with reduced power consumption.
✅ According to the World Health Organization (WHO), AI-powered MRI scans have improved diagnostic accuracy by 35% while reducing scan times by 50% (WHO, 2024).
✅ The Cleveland Clinic has implemented AI-optimized magnetic resonance imaging, reducing misdiagnosis rates by 27% (ClevelandClinic.org).
By leveraging AI-driven material design, hospitals can enhance imaging precision while cutting operational costs.
Study 2: Quantum Computing and AI-Driven Magnetic Field Concentrators
Quantum computers require stable and precisely controlled magnetic fields to function effectively. The Institut de Ciència de Materials de Barcelona’s flower-shaped microstructures offer a breakthrough in local magnetic field concentration, improving quantum coherence times.
✅ Google’s Quantum AI Lab reported that AI-optimized magnetic field concentrators have enhanced qubit stability by 45%, enabling more reliable quantum computations (Google AI Research, 2024).
This advancement is paving the way for ultra-powerful quantum computing applications in cryptography, drug discovery, and financial modeling.
Ethical Challenges and Future Innovations
Ethical Considerations in AI-Driven Materials Science
The integration of AI in magnetic metamaterials raises critical ethical questions:
Data Privacy: AI algorithms rely on vast datasets, including sensitive research information. Ensuring secure data handling is essential.
Bias in Material Predictions: AI models trained on biased datasets may overlook promising material innovations.
Environmental Impact: The production of advanced metamaterials must be assessed for sustainability, reducing resource-intensive manufacturing processes.
Future Predictions: AI and Magnetic Metamaterials in 2030
According to the World Economic Forum’s Future of Technology Report (2024), AI-powered metamaterials will drive major breakthroughs by 2030:
✅ Magnetic sensors will achieve near-zero energy consumption, reducing industrial power usage by 60% (WEF, 2024).
✅ AI-driven material discovery will accelerate the development of ultra-efficient superconductors, transforming the energy sector.
✅ Quantum computers powered by optimized magnetic microstructures will reach practical commercial deployment.
The combination of AI and magnetic metamaterials is set to revolutionize multiple industries, enabling more efficient, sustainable, and high-performance technologies.
Strategic Recommendations: Implementing AI-Powered Magnetic Technologies
To capitalize on the advancements in AI-driven materials science, organizations should adopt the following best practices:
📌 Best Practices for AI in Magnetic Metamaterials
✔ Invest in AI-Powered Simulations: Companies should leverage machine learning for material discovery to reduce R&D costs and accelerate innovation.
✔ Optimize Energy Efficiency: AI-driven magnetic field concentrators should be integrated into sensor technologies to minimize power consumption.
✔ Ensure Ethical AI Use: Organizations must implement bias-free AI models and prioritize transparency in material science research.
✔ Collaborate with Leading Institutions: Partnerships with AI and materials science research labs, such as those at MIT, Stanford, and ICMAB, will drive cutting-edge breakthroughs.
References & Further Reading
PwC, “AI Market Forecast 2024.” Available at: PwC
MIT Technology Review, “AI in Materials Science Report 2023.” Available at: MIT Technology Review
Harvard Business Review, “Ethical AI in Scientific Research.” Available at: HBR
Google AI Research, “AI and Quantum Computing Advances.” Available at: Google AI
For more expert insights on AI and emerging technologies, explore the research at 1950.ai and insights from Dr. Shahid Masood.
Comments