Can Meta Break Nvidia’s Grip on AI Hardware? A Deep Dive into the AI Chip Battle
- Dr Jacqueline Evans
- Mar 16
- 4 min read
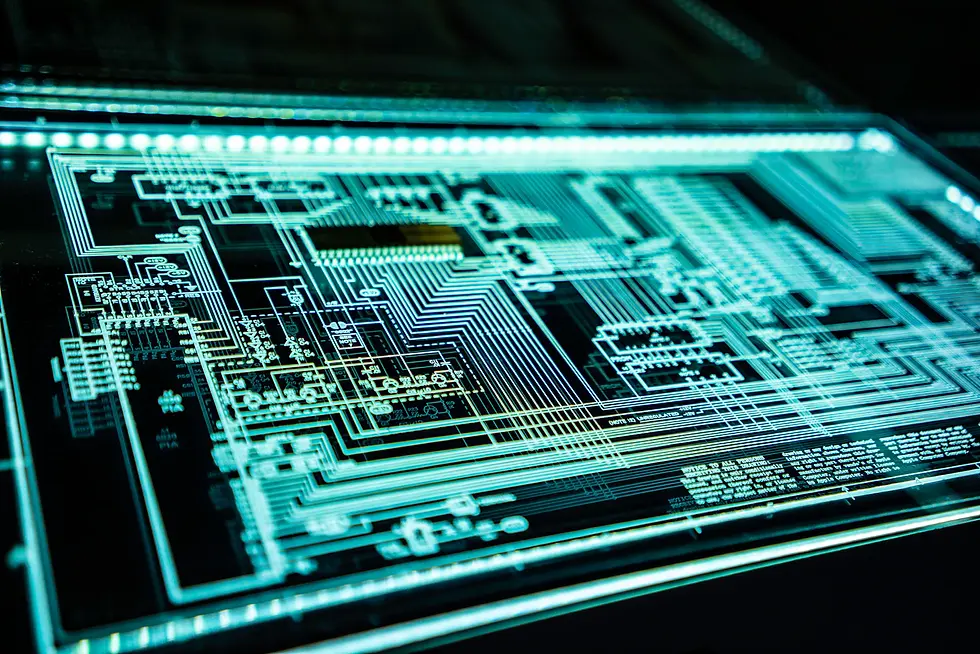
The artificial intelligence (AI) revolution has led to an insatiable demand for high-performance computing hardware, driving companies like Meta, Google, and Amazon to explore in-house AI chip development. With AI workloads becoming exponentially more complex, the reliance on Nvidia’s GPUs has put major tech firms in a precarious position—one where costs, supply chain bottlenecks, and strategic dependence on a single vendor could hinder future growth.
To counter this challenge, Meta has now developed its own AI training chip, marking a significant move towards self-sufficiency in AI hardware. This initiative follows Meta’s earlier ventures into AI inference chips, but now, with AI models like Llama 3 and beyond requiring even greater computational power, the company is pushing further to build custom silicon optimized for AI training.
But can Meta’s AI training chip truly compete with Nvidia’s dominant H100 and B200 GPUs? Or will this be another failed experiment in AI chip development? This article provides an in-depth analysis of Meta’s latest move, historical context, the technological advantages and limitations, financial implications, and the broader impact on the AI hardware market.
The Economics of AI: Why Meta Needs Custom AI Chips
Meta’s AI Infrastructure Spending: A Growing Concern
AI infrastructure has become one of the largest expenditures for companies developing large language models (LLMs), recommendation systems, and AI-driven applications. In 2023, Meta reportedly spent over $10 billion on AI-related infrastructure, primarily fueled by purchases of Nvidia’s AI GPUs.
This number is expected to rise sharply to $65 billion in 2025, with a significant portion allocated to Nvidia’s hardware. This raises a critical question: Is this level of expenditure sustainable?
Year | Estimated AI Infrastructure Spend | Estimated Nvidia GPU Spend |
2023 | $10 billion+ | $10 billion+ |
2024 | $50 billion+ | $20 billion+ |
2025 | $65 billion+ | TBD |
The Cost of Nvidia’s AI GPUs
Nvidia’s H100 and B200 GPUs are essential for AI training, but their prices make them a luxury that only tech giants can afford.
Nvidia GPU Model | Price per Unit (Estimated) | Power Consumption | AI Performance (TFLOPS) |
H100 | $30,000+ | 700W | 1,000+ |
H200 | $40,000+ | 800W | 1,200+ |
B100 | $45,000+ | 900W | 1,500+ |
B200 | $50,000+ | 1,000W | 1,800+ |
The high cost per unit, combined with rising energy consumption, makes the case for Meta to develop custom chips that are optimized for its AI workloads.
Meta’s AI Training Chip: A Deep Dive into the Technology
Meta has officially taped out its first AI training chip, a crucial step in semiconductor development that means the initial design has been finalized and is now in production. The company has partnered with TSMC (Taiwan Semiconductor Manufacturing Company), one of the world’s leading chip manufacturers, to fabricate the silicon.
Key Features of Meta’s AI Training Chip
Built on a RISC-V Architecture
Unlike Nvidia’s proprietary CUDA-based GPUs, Meta’s chip uses RISC-V, an open-source instruction set architecture (ISA) that allows complete customization.
This move eliminates the need for licensing fees associated with proprietary architectures like ARM and x86.
HBM3/ HBM3E Memory Integration
High Bandwidth Memory (HBM3) is a critical component in AI training as it allows massive data transfers between memory and the processor.
The use of HBM3E, the latest iteration, provides even faster speeds than standard GDDR6 or HBM2E used in Nvidia’s GPUs.
Systolic Array Architecture
Meta’s chip leverages a systolic array, a structured network of identical processing elements (PEs) arranged in a grid to optimize matrix operations.
This is crucial for tasks like backpropagation in neural networks, a key step in AI training.
Feature | Meta’s AI Training Chip | Nvidia H100 GPU |
ISA (Instruction Set) | RISC-V | CUDA (Proprietary) |
Memory | HBM3/HBM3E | HBM2E |
Processing Elements | Systolic Array | Tensor Cores |
Optimization | AI-Specific | General-Purpose AI |
Power Efficiency | High | Moderate |
This design means Meta’s chip is specifically optimized for AI workloads, whereas Nvidia’s GPUs are designed for broader use cases including gaming, rendering, and high-performance computing.
Meta’s AI Hardware: The Challenges and Risks
While the potential upside is significant, Meta’s AI chip journey is not without risks.
Performance vs. Nvidia’s GPUs
Nvidia has spent decades refining its CUDA-based AI hardware, making it an industry leader.
Early Meta inference chips failed to meet performance expectations—will the AI training chip suffer the same fate?
Supply Chain and Manufacturing Risks
Global semiconductor shortages have disrupted production timelines for major chipmakers, including TSMC and Samsung.
Can Meta scale production fast enough to replace Nvidia’s GPUs?
AI Model Evolution
AI models are growing at an exponential rate—from GPT-3’s 175 billion parameters to GPT-4’s estimated 1.5 trillion parameters.
Can Meta’s chip keep up with the rapid evolution of AI architectures?
The Bigger Picture: The AI Hardware Arms Race
Meta is not the only tech company developing custom AI chips. The AI hardware arms race is accelerating, with several major players investing in specialized AI silicon.
Company | AI Chip Initiative | Use Case |
TPU (Tensor Processing Unit) | AI training and inference | |
Amazon | Trainium & Inferentia | AI workloads on AWS |
Microsoft | Athena AI Accelerator | Azure AI services |
Apple | M-Series Chips | AI processing on mobile devices |
Meta | MTIA AI Training Chip | LLM training and recommendation AI |
The industry trend is clear: Tech giants want independence from Nvidia to control AI infrastructure and cut costs.
Meta’s AI Hardware Gamble – A Defining Moment
Meta’s decision to develop its own AI training chip represents one of the most ambitious moves in AI hardware. If successful, it could:
Reduce its reliance on Nvidia
Lower AI infrastructure costs
Optimize AI model performance
Set a precedent for RISC-V-based AI accelerators
However, challenges remain, from performance benchmarks to mass production scalability. The coming years will determine whether Meta’s AI chip becomes a groundbreaking innovation or another expensive miscalculation in AI hardware.
For expert insights into AI, cybersecurity, and emerging technologies, follow Dr. Shahid Masood and the expert team at 1950.ai. Stay ahead of the latest advancements shaping the future of artificial intelligence.
Comments