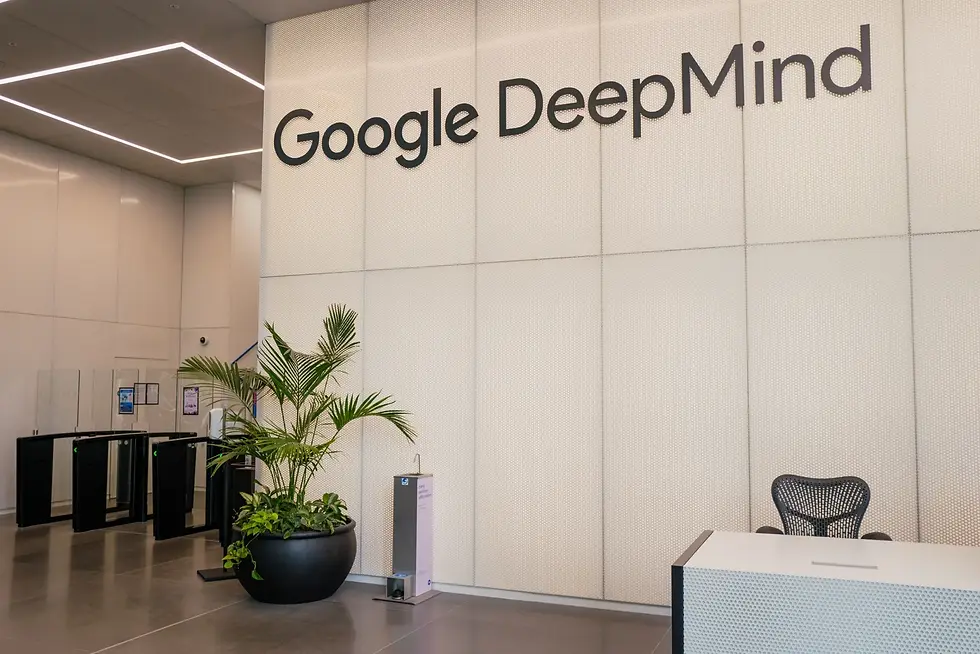
Artificial intelligence (AI) has rapidly transitioned from a conceptual technology to an essential tool transforming multiple sectors, including healthcare, drug discovery, and scientific research. One of the most promising areas in which AI is having a profound impact is drug discovery, a process traditionally hindered by lengthy timelines, enormous costs, and high failure rates.
Google DeepMind, a leading force in the AI revolution, is pioneering efforts to harness the power of machine learning to accelerate drug development, make it more efficient, and reduce the failure rate of clinical trials. Through its spin-off company, Isomorphic Labs, DeepMind aims to redefine the way drugs are discovered, developed, and brought to market, with AI playing a central role in this transformation.
In this article, we will explore the breakthroughs, challenges, and implications of DeepMind's work, as well as the broader potential for AI in drug discovery. From DeepMind's revolutionary AlphaFold system to its ambitious plans for the future of personalized medicine, we will delve into how AI is changing the landscape of healthcare and scientific research. By examining the practical applications of AI, the expected timelines, and the future of AI-driven scientific innovation, we can gain a better understanding of what lies ahead in the world of drug discovery and healthcare.
The Traditional Drug Discovery Process: Lengthy, Costly, and Unpredictable
Traditionally, the process of discovering and developing a new drug has been a time-consuming, resource-intensive venture. On average, it takes about 12-15 years for a drug to go from initial discovery to market approval. This timeline includes several phases, including target identification, preclinical research, and multiple stages of clinical trials. The financial cost of this journey is equally daunting, with estimates putting the cost of developing a new drug at around $2.6 billion.
Moreover, the success rate of drugs progressing through clinical trials is dismally low. Fewer than 10% of drugs that enter clinical trials actually make it to market, with many failing due to safety concerns or lack of efficacy. The high costs and risks involved have led to calls for new methods that can speed up the process, reduce the failure rate, and bring more effective drugs to market.
Traditional Drug Discovery Phases and Timeline
Stage | Duration | Success Rate | Key Challenge |
Discovery Phase | 6-8 years | 50-70% | Identifying viable drug targets |
Preclinical Research | 1-2 years | 40-50% | Ensuring safety and biological efficacy |
Clinical Trials (Phases I-III) | 6-8 years | 10-20% | Patient safety, dosing, and efficacy |
Total Time for Drug Development | 12-15 years | <10% | High failure rate, long timelines |
Average Cost | $2.6 billion | N/A | High financial burden on developers |
The traditional model has proven inefficient and unsustainable in the face of modern health challenges, including rising global health burdens, aging populations, and new emerging diseases. This has set the stage for AI to become a transformative force in the field of drug discovery.
DeepMind’s AI Revolution: AlphaFold and the Transformation of Drug Discovery
AlphaFold: A Groundbreaking AI Model for Protein Structure Prediction
One of DeepMind's most revolutionary breakthroughs in the field of healthcare is AlphaFold, an AI model that can predict the three-dimensional (3D) structures of proteins with remarkable accuracy. Proteins are the fundamental building blocks of life, and understanding their structure is crucial for designing drugs that can target specific diseases. Traditionally, determining the structure of proteins has been an arduous and time-consuming process, relying on techniques such as X-ray crystallography or cryo-electron microscopy. These methods can take years to complete and often yield incomplete or imprecise results.
However, AlphaFold has demonstrated the ability to predict protein structures with near-experimental accuracy. In 2020, the system achieved a significant milestone by solving the protein-folding problem—a challenge that has perplexed scientists for decades. AlphaFold’s ability to model protein structures accurately and efficiently opens up new possibilities for drug discovery. Scientists can now better understand how proteins interact with one another, how they function, and how they can be targeted by drugs.
Since its release, AlphaFold has been used to predict the structures of over 200 million proteins, with applications in understanding diseases such as Alzheimer’s, Parkinson’s, cancer, and COVID-19. This comprehensive protein structure database offers researchers invaluable insights into the molecular basis of disease and the potential for new drug targets.
Traditional vs. AI-Driven Protein Structure Prediction
Method | Traditional Methods | AlphaFold (AI-Driven) |
Time to Predict Protein Structure | 1-2 years | 1-2 days |
Accuracy | Moderate to Low | High (Near Experimental) |
Cost | High ($100,000 - $500,000) | Low (Free for academic use) |
Number of Structures Predicted | Limited | Over 200 million structures |
Data Requirements | Extensive experimental data | Trained on large protein datasets |
Isomorphic Labs: DeepMind’s Spin-Off for AI-Driven Drug Discovery
Building on the success of AlphaFold, DeepMind launched Isomorphic Labs in 2021, a spin-off company focused specifically on applying AI to accelerate drug discovery. The vision behind Isomorphic Labs is to use AI models to design drugs that can target specific diseases more efficiently. The company uses machine learning techniques to identify potential drug targets, predict how drugs will interact with proteins, and design new molecules optimized for disease treatment.
Isomorphic Labs leverages the same AI principles that powered AlphaFold but extends them to drug design. By analyzing large datasets, AI can predict how molecules will interact with protein targets associated with diseases, speeding up the early-stage drug discovery process. This AI-driven approach has the potential to significantly reduce the time and cost required to develop new drugs, making treatments more accessible and affordable.
AI-Designed Drugs Entering Clinical Trials by 2025
DeepMind’s CEO, Demis Hassabis, has made bold predictions about the future of AI in drug discovery. During a panel discussion at the World Economic Forum (WEF) in January 2025, Hassabis revealed that AI-designed drugs could begin clinical trials as early as 2025. According to Hassabis, the goal is to have AI-designed drugs in clinical trials by the end of this year, marking a significant milestone in the field of healthcare.
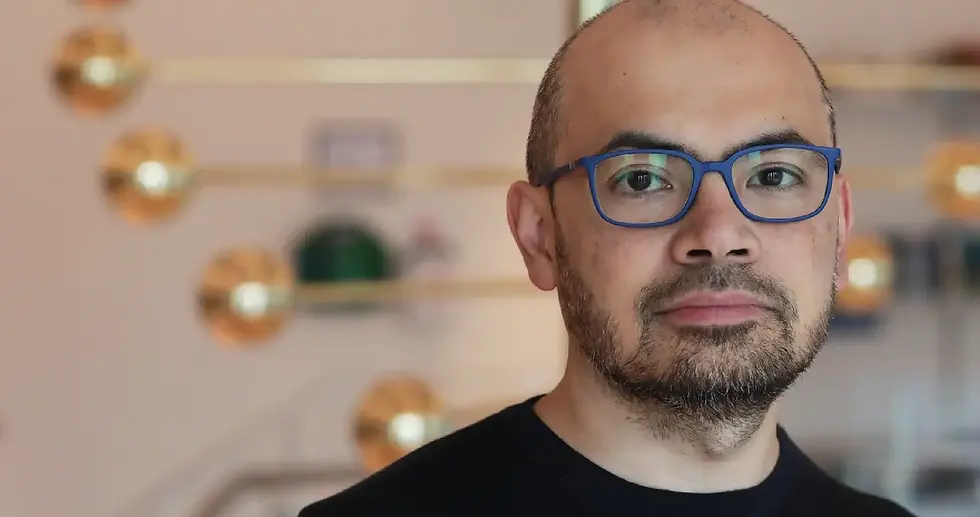
The timeline for AI-designed drugs entering clinical trials is ambitious, but it is grounded in the progress already made in AI-driven drug discovery. With advancements in AI algorithms, the use of synthetic data, and collaborations with clinical research organizations, DeepMind is confident that its AI models will be able to accelerate the development of viable drug candidates that can move through the clinical trial process faster and more efficiently.
AI in Drug Discovery: From Faster Development to Cost Reduction
The ability of AI to analyze massive datasets and predict the effectiveness of potential drug candidates could transform the drug discovery pipeline. By leveraging AI, researchers can:
Design Drugs More Quickly: AI algorithms can process vast amounts of data and design molecules optimized for specific biological targets in a fraction of the time it takes traditional methods.
Reduce the Failure Rate: AI can predict the likelihood of success or failure of drug candidates early in the process, allowing researchers to focus on the most promising molecules and avoid wasting resources on ineffective drugs.
Cut Costs: AI-driven drug discovery can reduce the costs associated with early-stage drug development, as AI models can predict molecular interactions and drug efficacy before human trials begin, reducing the number of compounds that need to be tested in vivo.
The economic impact of AI in drug discovery cannot be understated. If successful, AI-driven methods could reduce the cost of developing a new drug by as much as 80%, saving billions of dollars and years of research. This would allow for more drugs to be brought to market, particularly in therapeutic areas where research funding is limited.
The Financial Impact of AI in Drug Discovery
Metric | Traditional Drug Discovery | AI-Driven Drug Discovery |
Average Cost to Develop a Drug | $2.6 billion | $500 million - $1 billion |
Time to Market | 12-15 years | 3-5 years |
Failure Rate in Clinical Trials | <10% | 30-50% |
Number of New Drugs per Year | 40-50 | 100+ |
Ethical and Regulatory Considerations in AI-Driven Drug Discovery
While the promise of AI in drug discovery is immense, there are significant ethical and regulatory challenges that must be addressed. These include:
Data Privacy and Accessibility
AI systems require vast amounts of high-quality data to function effectively. In healthcare, this often means accessing patients' genetic data and medical records, raising concerns about privacy and data security. Regulatory frameworks will need to evolve to ensure that AI models are trained using diverse and representative datasets while protecting patient privacy.
Accountability and Liability
As AI systems become more autonomous, questions about accountability will arise. If an AI-designed drug causes harm to a patient, who is responsible? Ensuring that AI in drug discovery remains transparent and accountable will be crucial to its widespread adoption in the medical field.
The Future of AI: DeepMind’s Vision Beyond Drug Discovery
Demis Hassabis has outlined an ambitious vision for the future of AI, one that extends beyond drug discovery. DeepMind is working on projects aimed at using AI to solve some of the world’s most pressing challenges, including climate change, quantum computing, and personalized medicine. By creating AI systems that can understand and predict complex biological processes, DeepMind hopes to unlock new frontiers in science and healthcare.
“The true potential of AI is not just in automating existing tasks, but in enabling entirely new kinds of scientific discovery.” — Demis Hassabis, CEO of DeepMind
As AI continues to evolve, we can expect it to play a growing role in medical research, scientific innovation, and the future of healthcare. With AI's ability to analyze massive datasets, predict molecular interactions, and design targeted therapies, the possibilities are endless. The promise of AI in drug discovery is just the beginning; its potential to revolutionize science, healthcare, and society is vast.
The AI-Driven Future of Drug Discovery
DeepMind's work in AI-driven drug discovery represents a monumental leap forward in scientific innovation. Through the development of powerful AI models like AlphaFold and the establishment of Isomorphic Labs, DeepMind is poised to transform the way drugs are discovered, designed, and brought to market. By reducing the time, cost, and failure rate of drug development, AI has the potential to not only save lives but also reshape the global healthcare landscape.
While challenges remain, particularly around ethical considerations and regulatory approval, the future of AI in drug discovery is undoubtedly bright. As we look ahead, we can expect more AI-designed drugs entering clinical trials, with the promise of a more efficient, cost-effective, and personalized approach to healthcare.
In the coming years, AI could become the backbone of drug discovery, ushering in an era where treatments for previously untreatable conditions become a reality. With AI’s ability to analyze, predict, and design, the future of healthcare looks poised for transformation.
For expert insights into the future of AI in drug discovery and healthcare, follow Dr. Shahid Masood and the expert team at 1950.ai.
Comments